Decades ago, in response to mounting concerns within IBM regarding public apprehension surrounding the company’s commercial presence and its work for the U.S. Department of Defence, a concerted effort was made to reshape public opinion and foster a more personal connection with the computer. To achieve this goal, Charles and Ray Eames were commissioned to create films that would humanize the computer. The outcome was the 1958 short film in which the Eameses aimed to present the computer as a tool that aids humankind. The film sought to establish a connection between computing devices and human thought, emphasizing the potential benefits and assistance offered by this technology. Years later, the couple created the film Powers of Ten (1977), an exploration that traverses both the microscopic and macroscopic realms. This work presents a deceptively simple yet seamlessly interconnected universe, showcasing IBM’s technological abilities.
Much like the Eameses’ project from years gone by, and countless more recent examples, we witness the effectiveness of the arts as a means to showcase technology, acquaint people with advancements, and perhaps even unveil their underlying mechanisms. However, more importantly, art also possesses the capacity to criticize new developments, to highlight instances where technology falls short and doesn’t work as promised – at least not for marginalized groups of people.
These days, the interplay between human and machine prompts philosophical questions around what it means to be human and what it means to be machine? Undoubtedly, recent months have borne witness to transformative shifts in how AI shapes the realm of arts and culture. We have seen dynamic collaborations between artists and machines, AI playing roles in the digital conservation of artistic heritage, and the seamless integration of augmented and virtual realities into museum and gallery encounters. Furthermore, AI’s influence extends to spheres like content curation, as seen on platforms like Spotify and Netflix where data-driven analyses foster tailored recommendations. In the most recent developments, we’ve observed advancements in generative artificial intelligence, like Midjourney and Dall-E. These systems possess the capability to craft images based on user prompts.
In his book The Black Technical Object, the visual and cultural theorist Ramon Amaro reminds us of the recurrent cycles of “racialized information,” by which he means a set of atmospheric conditions that organize humans and technical objects around assumptions of race. 1 In fact, the evolution of machine learning is conditioned by such factors. He describes a negation of black existence by the machine, which is set for an algorithmic version of a white imaginary. Therefore, it is essential to understand how a machine functions, which can be comprehended on different levels. The first, which often proves challenging for most individuals, involves delving into the technical, mathematical, and coded language that underpins it. The second level focuses on its operational mechanism: what does the algorithm learn from and how do these insights interconnect with other technological frameworks and systems? An effective approach to grappling with the complexities of machine learning requires a multidisciplinary stance, encompassing not only technical perspectives, but also critical discourse and visual art. Artists have taken a pioneering role in addressing, confronting, and portraying the intersections and shared concerns among various realms of research, rendering them accessible to a broader audience.
- Ramon Amaro, The Black Technical Object: On Machine Learning and the Aspiration of Black Being (MIT Press, 2023).
The artist Stephanie Dinkins is a pioneer in working with art and AI, who highlights social engagement, transparency, representation, and equity in her work. For the past seven years she has examined AI’s ability to make a realistic representation of a Black Woman crying and laughing, by using a whole range of word prompts. Yet, the outcome of her efforts is puzzling: a humanoid in shades of pink, draped in a black cloak, revealing to us the software’s inability to follow a seemingly simple prompt. Dinkins’ work reminds us that racial bias remains deeply entrenched within machine learning. To what extent is the complex entanglement between AI and bias rooted, and how might artists shed light on these issues?
Let’s begin by delving into the functioning of artificial intelligence (AI). Machines endeavor to reflect our society, yet their mode of operation differs considerably from human cognition. While human thinking involves consciousness, emotions, and intricate subjective experiences, machine thinking is based on algorithms and data processing. Artificial intelligence takes input data and transforms it into a different format through the application of algorithms—essentially a set of predefined rules or instructions shaped by mathematical computations. Subsequently, the AI employs pattern recognition to anticipate potential outcomes, drawing on patterns learned from data. For decision-making, the AI follows predetermined rules and instructions, arriving at a conclusion. Therefore, the bedrock of an AI’s comprehension and decision-making lies in its capacity for classification and categorization.
It is important to acknowledge that the visual classification of humans is not confined to AI practices – rather, these new technologies are extensions of a historical trajectory. Throughout history, the categorizations of genders, races, sexualities and so on have been widely scrutinized in different disciplines. It is clearly evident that assigning individuals to rigid categories has resulted in tangible harm. The Jamaican novelist and philosopher Sylvia Wynter underscores the power and production of knowledge as a principal weapon by which difference is formed, and then reiterated. According to Wynter, the concept of “race”, despite lacking anatomical foundations, has been instrumental in allowing the Western world, in the midst of global expansion, to replace prior categorizations such as mortal/immortal, natural/supernatural, human/ancestors. These former distinctions had underpinned diverse human groups' perceptions of humanity, but they have now been supplanted by a fresh differentiation between the human and the subhuman. 2
- Sylvia Wynter, “Unsettling the Coloniality of Being/Power/Truth/Freedom: Towards the Human, after Man, Its Overrepresentation--an Argument,” CR: The New Centennial Review 3, no. 3 (2003): 257–337, https://doi.org/10.1353/ncr.2004.0015.
Denise de Ferreira da Silva also traces the notions of racial and cultural difference back to at least post-enlightenment Europe; the figurings of determinacy and ethics served as a foundation for modern thought, and the very tools of scientific reason were deployed to account for human difference. As the European/White mind alone shared a key quality with universal reason, a differentiation between white and other races was perceived necessary. 3 The grouping together of people and the establishment of differences led to the further actualization of those differences. The naming of races, such as the Caucasian, the oriental, the negro, and the use of these terms as a basis for colonization, settlement, and enslavement, led to economic transformation and new social configurations. Is the default whiteness we see in the development of AI technologies today the perpetuation of these traditions?
- Denise Ferreira da Silva, “1 (Life) ÷ 0 (Blackness) = ∞ − ∞ or ∞ / ∞: On Matter beyond the Equation of Value,” E-Flux Journal, no. 79 (2017).
In the Archaeology of Knowledge, Foucault called for an archaeological methodology, to dig into the systems of knowledge, examine how knowledge circulates in and transforms society, and not least consider how these systems shape social categories. 4 There is a need for an examination of the invisible forces that preserve these systems. In their book Sorting Things Out: Classification and its Consequences, Geoffrey C. Bowker and Susan Leigh Star emphasize the role of invisibility in classification – how categories are made and kept invisible and which power mechanisms are at play. 5 Their work examines how every standard or category carries inherent biases, privileging certain perspectives while suppressing others. This duality isn't inherently negative; its ethical judgment depends on contextual factors and the power dynamics at play. What remains undeniable is its inescapability. Consider, for instance, the classification of students based on standardized test scores and aptitude assessments. Such categorization inherently favors particular forms of knowledge and competencies while neglecting others. Consequently, certain skill sets remain concealed within this framework. In this light, classifications yield a dual impact: they can either unlock opportunities or deprive individuals of them. The consequence hinges on how these classifications align with societal values and power structures.
- Michael Foucault, The Archaeology of Knowledge (London: Routledge, 1969).
- Geoffrey C Bowker and Susan Leigh Star, Sorting Things Out: Classification and Its Consequences (Cambridge, Mass.: MIT Press, 2008).
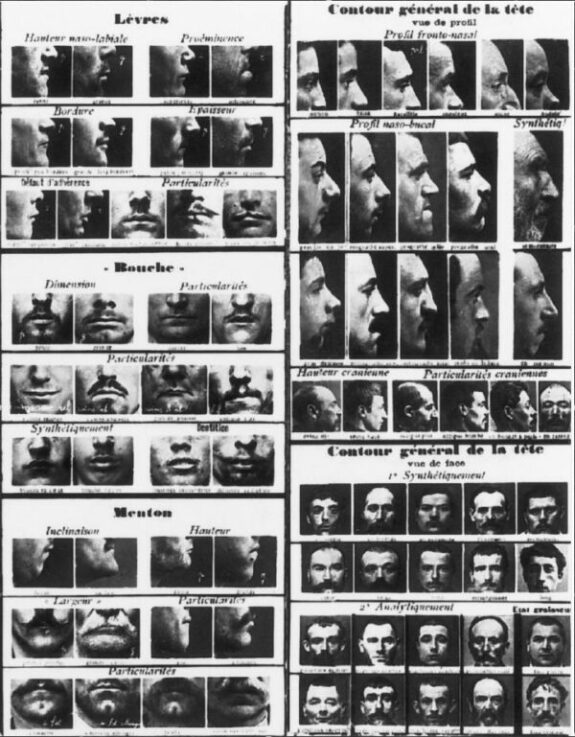
Visual technologies are intricately linked to classification systems due to their role in perceiving, organizing, and interpreting the visual world. These technologies enable capture, analysis, and representation of visual information, which can then be categorized and classified based on various criteria. In "Body and the Archive," Allan Sekula highlights a historical instance where screen media and classification practices are intertwined. 6 The advent of photography marked the inception of visual documentation practices. Photographic portraiture, initially serving medical illustration purposes, ended up shaping both the standardized appearance, or typology, and instances of divergence, such as cases of social deviance. This interplay between photography and classification was particularly evident in the context of criminal identification.
- Allan Sekula, “The Body and the Archive.”, October 39 (1986): 3–64.
In the pursuit of defining a criminal profile and establishing a comprehensive criminal database, pivotal figures emerged in the French and English police forces, namely Alphonse Bertillon and Francis Galton. They harnessed the methodologies of physiognomy and phrenology—taxonomic disciplines grounded in the belief that an individual's inner character could be discerned from the external features of the body, notably the face and head. Their aim was to encapsulate the entirety of human diversity within an archive specifically dedicated to criminals. 7 However, their influence extended far beyond interpretation, as they played an important role in constructing the very archive they purported to merely decode. They accomplished this by transforming the human body into an indexical repository of data, meticulously comparing and interpreting binary facets to chart human attributes. This classification process was underpinned by a repressive logic that juxtaposed qualities like genius, virtue, and strength with their perceived opposites—idiocy, vice, and weakness.
- Allan Sekula, “The Body and the Archive.”, October 39 (1986): 3–64.




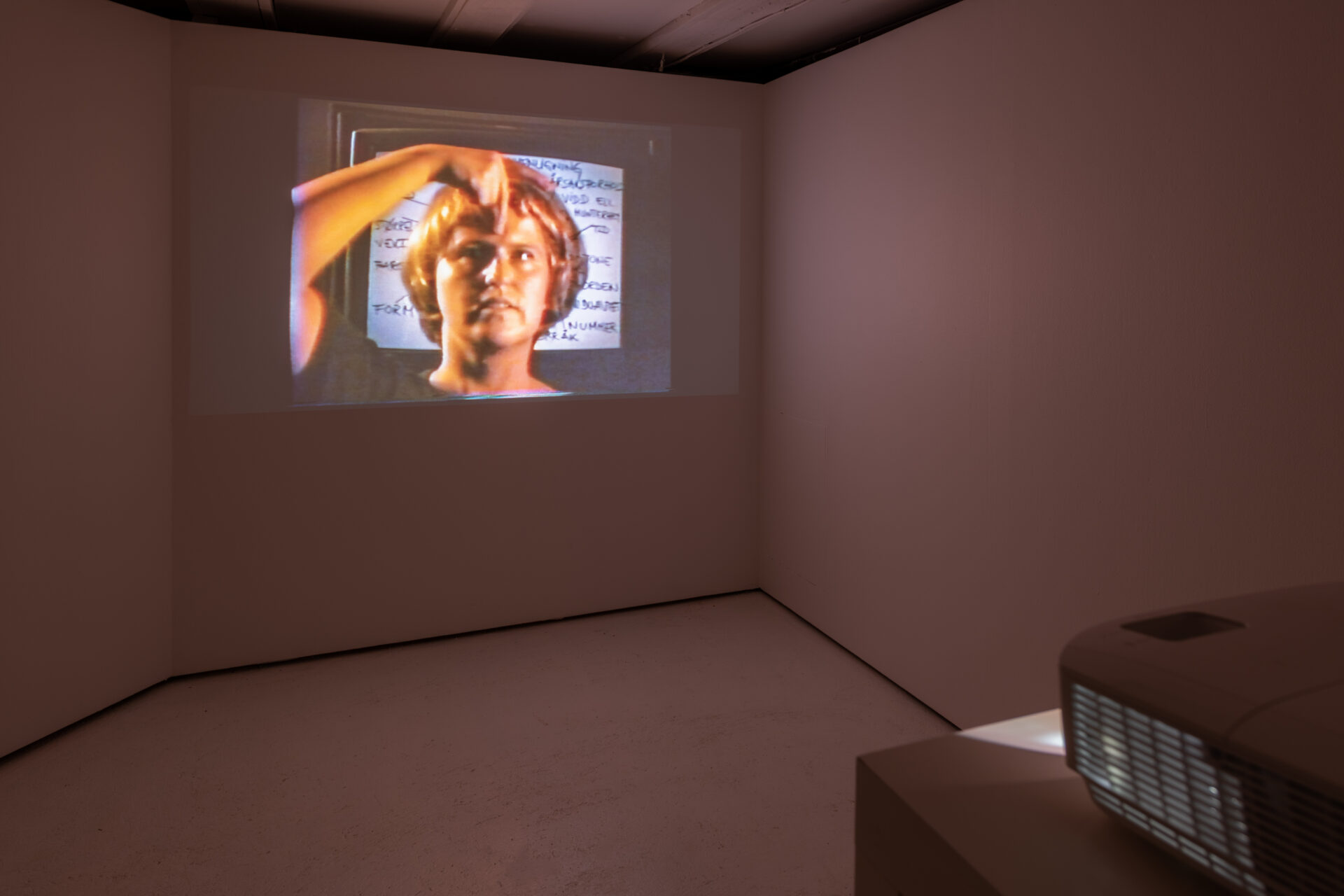
Physiognomy, for instance, ascribed distinctive characterological meanings to every component of the head, from the forehead to the eyes, ears, and nose. Phrenology, on the other hand, forged links between cranial features and cerebral attributes. These methodologies operated on the premise of assigning profound implications to physical marks, engendering a system with tangible consequences. Such practices still exist today. The Norwegian artist Marianne Heske made a photo series in the 1970’s titled Phrenological Photo Analysis, which was re-exhibitied in 2020 in an exhibition titled Artificial Intelligence at QB gallery in Oslo. In the accompanying exhibition text, Ivo Bonacorsi writes: “Probably the roots of the many formats of our digital era lay in those false phrenological ruminations about the shape of the human skull or in these old physiognomy studies. Even a false science was capable of providing mapping of needs that fulfilled feelings, emotions, attitude.” An algorithmic and reductive processing of human typology long precedes AI tools.
An algorithmic and reductive processing of human typology long precedes AI tools.
Photo identification was misused in the cruellest ways during the apartheid regime of South Africa. A mix of confused pseudo-scientific practices and eugenics along with the appropriation of anthropological studies was employed to justify the exertion of violent power. Racial classifications, materialized in a “racial pass,” determined where people could live, move, and work. A person’s racial category determined their level of political rights and freedoms, which meant that those categorized as white experienced obvious benefits. Apartheid rule lasted for four decades during which people were arrested, murdered, and exiled for not existing within their prescribed limits.
The scientific reason given by the white supremacist party was that to develop naturally, different races must develop separately. Yet, the definition of race, and racial categories has yet to be scientifically defined and the differentiation processes of the system were not as seamless as expected by some. Despite a legal requirement for certainty in race classification, many people did not conform easily to the constructed categories. Some people had appearances that differed from the assigned category, or they lived with someone of a different race, or spoke a different language than their group. There were constant inconsistencies and local work-arounds to sustain the lawful categories. More than a 100,000 people made formal appeals regarding their race classification and reclassification often turned into a Kafkaesque process where most of the applications were denied. The classification practice was mostly based on visual observation and assessment, so dark-looking children with a “white pass” could still be rejected from a white school. Diversity within a race category, such as language and cultural practices, were often discarded in favour of racial differentiation. The philosopher-psychiatrist Franz Fanon argued that Blackness as an identity is constructed and produced – locking black people into blackness (and white people into whiteness) as a colonized psychic construction. 8
- Frantz Fanon, Black Skin, White Masks, trans. Charles Lam Markmann (1952; repr., London: Pluto Press, 1986).
Blackness as a category has thus always been visual, about skin colour, and when absorbed by machine learning algorithms, the outcomes are still harmful. Achille Mbembe writes: “Recent progress in the domains of genetics and biotechnology confirms the meaninglessness of race as a concept. Paradoxically, far from giving renewed impetus to the idea of a race-free world, they are unexpectedly reviving the old classificatory and differentiating project so typical of the previous centuries.” 9
- Achille Mbembe, Necropolitics (Durham: Duke University Press, 2019), 180.
We can understand machine learning on several levels: one is harder for most people: the technical, mathematical, coded language. Yet, the other is how it functions – what does the algorithm learn from and how does this relate to other technologies and systems? The classification process in machine learning involves several steps: Firstly, the data needs to be appropriately represented in a format that the machine learning algorithm can work with. For example, in image classification, images might be represented as arrays of pixel values, 10 while in text classification, textual data might be converted into numerical vectors through techniques like word embeddings. Then relevant features are extracted from the data to represent its characteristics. These features act as the input that the algorithm uses to make predictions. In supervised learning, the algorithm is provided with a labeled dataset where each data point is associated with a corresponding class label. The algorithm learns to map the features of the data to the correct class labels by iteratively adjusting its internal parameters through optimization techniques. During training, the algorithm learns to identify decision boundaries that separate different classes.
- A pixel, short for picture element, is the smallest addressable (possibly manipulatable) element in an image. Each pixel in a colour image has an intensity, a colour, represented by numerical values (by three components in the RGB colour space or four in CMYK and so on).
Once the model is trained, it can be used to classify new, unseen data points. The input features of the new data are fed into the trained model, which applies the learned decision boundaries to predict the class label associated with the input. Which means that it’s important to note that the effectiveness of a classification algorithm depends on various factors, including the quality and representativeness of the training data, the complexity of the underlying patterns, the choice of algorithm, and so on. Biases present in the training data will therefore impact the algorithm’s classification decisions.
Biases present in the training data will therefore impact the algorithm’s classification decisions.
So, when does the misidentification of the incoming image actually occur? To fully grasp this, a more in-depth examination of the training sets becomes necessary. One of the most prominent examples in recent research is the training dataset known as ImageNet. ImageNet didn’t emerge from thin air; it has a lineage that connects it to previous classification systems. The categorization within ImageNet maintains taxonomical hierarchies inherited from its predecessors, encompassing categories that span beyond the realm of machines. The majority of these categories are derived from its precursor, WordNet, which itself has roots in the Linnean system of biological classification and the organization of books in a library. Upon closer analysis, the underlying politics of classification become notably apparent
ImageNet has a collection of 21,841 categories, with a significant portion of them being innocuous. However, fixed categories inherently lack the capacity to perceive the subtle nuances and multiple connotations inherent to given nouns. Consequently, the spectrum of ImageNet's categories becomes flattened, causing valuable information residing between these defined labels to vanish. Initially, ImageNet contained a staggering 2,832 subcategories dedicated to "person," spanning attributes such as age, race, nationality, profession, socioeconomic status, behaviour, character, and even morality. Even seemingly benign classifications like "basketball player" or "professor" carry a plethora of assumptions and stereotypes, encompassing aspects like race, gender, and ability. Hence, neutral categories remain elusive, as the training datasets employed in AI inherently encapsulate a particular worldview, which subsequently influences the generated output. As pointed out by the researcher Kate Crawford: “While this approach (of ImageNet) has the aesthetics of objectivity, it is nonetheless a profoundly ideological exercise.” 11
- Kate Crawford, Atlas of AI: Power, Politics, and the Planetary Costs of Artificial Intelligence (Yale University Press, 2021), 184.
Even seemingly benign classifications like “basketball player” or “professor” carry a plethora of assumptions and stereotypes, encompassing aspects like race, gender, and ability.
In 2019, Crawford and the artist Trevor Paglen made a collaborative project titled ImageNet Roullette. Developed as a part of their exhibition Training Humans at Milan’s Fondazione Prada, the website’s primary objective was not to employ technology to aid us in understanding ourselves, but rather to utilize our own perspectives to gain insights into the true nature of technology. The algorithm powering the website underwent training using images from the ImageNet database which consists of more than 14 million photographs.
Should you choose to upload your own photograph, ImageNet Roulette employs AI to detect any present faces and subsequently assigns them labels from among the 2,833 subcategories representing different types of individuals within ImageNet's comprehensive classification system. For example, a technology journalist in the Guardian, with Asian background, was assigned racial slurs. 12 This was exactly the outcome that Paglen and Crawford intended, thus revealing the flawed training data set. Days after the digital art project went viral, and people complained about the highly problematic outcome of the project, ImageNet released a statement saying that it will remove 438 people categories and 600,040 associated images that they have now labeled as unsafe. 13 This solution echoes what Bowker and Star observed in previous classification practices: “politically and socially charged agendas are often first presented as purely technical and they are difficult even to see. As layers of classification system become enfolded into a working infrastructure, the original political intervention becomes more and more firmly entrenched. In many cases, this leads to a naturalization of the political category, through a process of convergence. It becomes taken for granted.” 14
- Julia Carrie Wong, “The Viral Selfie App ImageNet Roulette Seemed Fun – until It Called Me a Racist Slur,” The Guardian (The Guardian, September 18, 2019), https://www.theguardian.com/technology/2019/sep/17/imagenet-roulette-asian-racist-slur-selfie.
- News Desk, “Image Database Purges 600k Photos after Trevor Paglen Project Reveals Biases,” Artforum, September 24, 2019, https://www.artforum.com/news/image-database-purges-600k-photos-after-trevor-paglen-project-reveals-biases-80829.
- Geoffrey C Bowker and Susan Leigh Star, Sorting Things Out: Classification and Its Consequences (Cambridge, Mass.: MIT Press, 2008), 196.
Stephanie Dinkins made a more defined and personal attempt at making a similar point with several of her works. The ongoing project Not The Only One (N’TOO) is a multigenerational memoir of a black American family told from the perspective of an AI. This ever-evolving project feeds the data set with data that the artist has chosen herself and watches the intelligence grow. In another project titled Conversations with Bina48, Dinkins presents recordings between herself and the humanoid Bina48. The project is described as a quest for friendship with a humanoid robot turned into a rabbit-hole of questions about the future and an examination of the codification of social, cultural and future histories at the intersection of technology, race, gender and social equity.
The harmful consequences of racial bias in AI stretch way beyond hypothetical situations and purposefully constructed harms in art projects. For example, a couple of years ago, a facial recognition tool wrongly identified an American-born university student and Muslim activist as a suspect in the Sri Lanka Easter bombings. Amara K. Majeed, a daughter of Sri Lankan immigrants, had her image released by the authorities and included in the media coverage of the killings. Even if the misidentification was later corrected, the harm was already done and Majeed received countless death threats, and her family was put in danger. A now much-quoted MIT study proved that facial recognition technologies have an error rate 49 times higher for dark-skinned females than for white males. 15 Most alarmingly, this technology is already implemented in society, despite its proven fundamental danger to certain groups of people. 16 Black and brown people are subjected to discrimination and suspicion through everyday activities and transactions such as browsing the internet, applying for jobs and using a credit card.
- THE FOOTNOTE TEXT
- THE FOOTNOTE TEXT

American Artist has made several works that comment on the role of machine learning and digital networks in surveillance and policing. Veillance Caliper (annotated) (2021) is an open cube-shaped plank construction which represents a three-dimensional graph. There are measurement marks along the planks and when you look closely, annotations have been written in several places such as “(z) dark?,” “cctv,” “racially saturated,” “ant-sousveillance” and so on. Even if the structure is open, once the hidden message is decoded, it feels like a closed space, hard to escape. Such, American Artist points to the role of surveillance technologies in the racist policing in the US. Facial recognition, the attaching of numerical values to the human face, is scarily similar to what Bertillon and Galton did with phrenology and physiognomy. By virtue of the way they work on a technical level, facial recognition softwares reinforce discredited categorizations. In fact, it seems like the moral compass of facial recognition technologies and other image recognition practices in AI are modelled after more primitive modes of categorizations of human faces, rather than being informed by the many years of scholarly critical work on these categories.
Years ago, when IBM commissioned Charles and Ray Eames to create videos and exhibitions, the goal was to show how intelligent the machine is. Yet, artists like American Artist, Trevor Paglen and Stephanie Dinkins have shown us the limitations and harmful outcomes of machine learning – how unintelligent the machine can be. We have seen how most algorithms are designed for recognition and reinforcement, and thus reinforce existing ideologies and biases in society. It is essential to acknowledge the historical context and ethical implications woven into the development and application of AI and visual technologies. The path forward must involve critical examination, multidisciplinary collaboration and not least the contribution of visual arts that reveal both the potential and pitfalls of technology.
Abirami Logendran is a writer and graphic designer. She works as a film programmer for Kunstnernes Hus Cinema and is a film critic for Klassekampen and various magazines. With three bachelor degrees in mathematics, history and graphic design, as well as a masters in screen cultures from University of Oslo, she is currently finishing her masters in fine arts at Oslo National Academy of the Arts.